There’s a lot of buzz about AI these days, and technologies like Deep Natural Networks (DNN) or Convolutional Neural Networks (CNN) are often mentioned in the news. While these techniques are great for image classification and speech recognition applications, they don’t work very well on time-series data that is the basis for trendline forecasting, anomaly detection, and predictive maintenance. Traditional reactive and fixedschedule approaches to maintenance and quality control are no longer yielding significant improvements, and companies must adopt AI technologies in order to get to the next level. Most companies lack the much needed resources and expertise to tackle the problem, so it often takes a back seat to firefighting activities. That’s where Tangent Works really shines. The company’s solutions can analyze historical data from equipment and sensors to spot anomalies and cause-effect relationships that can be used to optimize maintenance cycles and avoid unplanned downtime.
Predictive models
Tangent Works was founded in 2014 by a team of data scientists and mathematicians who believed in the power of predictive modeling for optimizing operations and reducing costs, but found that the existing methods were far too complex for broad adoption. The company is focused on developing a technology that automates the process to create predictive models for forecasting and anomaly detection. The company’s InstantML technology creates a model in seconds based on target and predictor series with one pass through the data. With RTInstantML (Real-Time Instant ML), TangentWorks goes one step further. The speed of the model generation process allows them to model/predict in one go. Building and refining accurate predictive models was a laborious, iterative task that required a combination of a domain and data science expertise and weeks/months of effort.
TIM, Tangent Information Modeler, is a predictive modeling engine that automates the forecasting and anomaly detection processes by analyzing time-series data and generating accurate models on the patterns it detects. In just few minutes TIM creates a predictive model ready for validation and deployment. It automates the model generation process so one can create and update predictive models without being an AI expert. It automatically creates predictive models for each asset, customer or group of customers that can accurately forecast consumption for the days/weeks/months ahead. It provides traders with an accurate predictive model and an understanding of the dynamics of price variability.
Use of Massive Computing Resources
The need for automated Machine Learning solutions is widely recognized. Big players have to build Auto ML functionalities. They do this by using massive computing resources to calculate many potential models and later select the most performing ones. This strategy works partially. It reduces engineering time, but it remains an engineering task. For large scale consumption meaning areas that need a lot of individual models, the required computing resources and engineering time form a burden. Tangent took another mathematical approach to the problem. It makes that the company construct the features and the models from the ground up resulting in fast model generation with limited computing resources. This allows for large scale automatic time series forecasting and anomaly detection.
Integrate cutting-edge Capabilities
Tangent’s goal is to complement the existing data science platforms and make them better, faster, cheaper, and with more impact on time series data. To achieve that goal, the company partners with organizations like Microsoft, Alertyx to integrate its cutting edge capabilities so that its clients can benefit from the company’s research. Years of research have created the TIM engine based on a blend of various technologies, including Information Criteria / Geometry.
Many companies offer AUTOML capabilities to support the model generation engineering process. These tools/platforms allow you to build, tune, compare models, test the models, deploy the selected model as an API to production. AUTOML offers a step forward but it remains time-consuming expertise intensive and thus less scalable.
Sharp and Visionary Leader
Henk De Metsenaere is the Co-Founder of the company. His engineering background was complemented with a degree in economics. That made him worked in technical commercial jobs. The larger part of his career he worked as a regional sales and marketing director for SAP, a global software company. In that role, he learned that technology and sometimes interesting concepts only get adopted when they become digestible for larger audiences. He felt there was a gap between the hype around machine learning and the reality in companies that want to implement these ideas.
For Henk, the future of machine learning is all about mass scaling in many areas of the business and operations. Just as first cars were produced one by one and then later got produced on automated lines to fulfill the mass mobility need, Henk believes that the same is happening with Machine Learning. All the initial objections of experts are there, fear for jobs, rumors on lack of quality. The same things happened in the car industry. ‘But just as modern cars are better and more engineers work in the industry than ever before, also the objections in the current ML market are not real. It is just the scale that will change. Quality is there, people will continue to work in this industry only what they do will be different but will allow for mass consumption of these wonderful ideas’.- asserts Henk
Performing Predictive and Prescriptive Analytics
Machine Learning is not new. Many concepts were developed in the 40-50’s of the last century. After the AI winter of the 1970s, back-propagation as a mechanism to efficiently train neural networks gave rise to new cases. Machine Learning is now a subset of AI that allows computer algorithms to learn from data and information and perform predictive and prescriptive analytics. A wide variety of modeling techniques are available. The algorithms/models need a careful selection of data to be used as well as proper feature engineering. This still makes it a hard task to do.
Future with Machine Learning
The future of machine learning will depend on the degree that companies can scale available resources so the concepts can be implemented easier and with more results. The initial founders wanted to simplify the use of machine learning (ML) models in a business environment. The company brings ML in a time series context from the expert corner to the business user. This results higher adoption rates of promising ML ideas in operational business scenarios. The company found that time series modeling is quite complicated but if solved it can add a lot of value to a wide variety of challenges. The outcome is TIM, Tangent Information Modeller – A modeling engine reducing hours and days of costly engineering, into seconds of computing time.
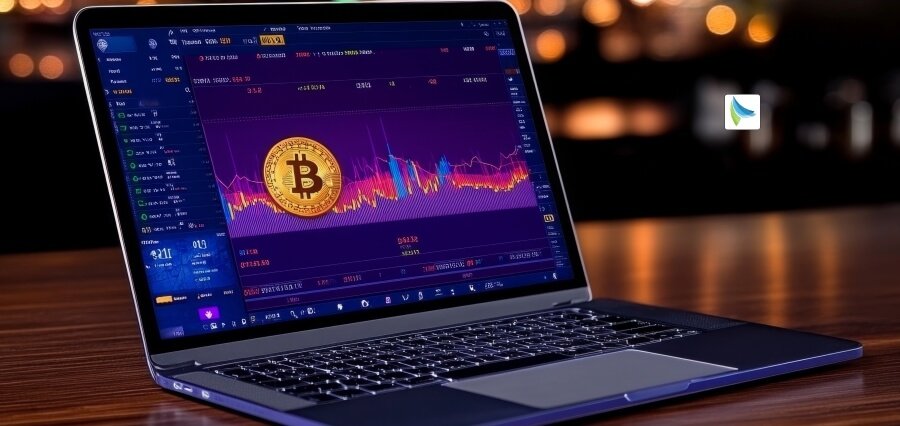